Juniper Publishers- Open Access Journal of Case Studies
Real World Data Hub-A Roadmap for Comprehensive Data Strategy and Evidence Development November 17, 2017
Authored by Frank Corvino
Mini Review
The evidence required to support pharmaceutical and medical device products is undergoing a historic evolution driven by the increasing rigor, proliferation, and use of Real World Data (RWD). This ubiquity of RWD is ushering in an era of evolving policy, regulation, and value assessment which will help patients, providers, and payers make appropriate treatment decisions.
Pharmaceutical and Medical device manufacturers have an opportunity to streamline the development of evidence and insights from RWD by developing internal Data Science capabilities. When executed correctly, this internal Data Capability or "Data Hub" is a systematic structure for delivering evidence support to internal, cross functional departments with a service-provider mentality.
The key components of the Data Hub include:
a) Data Governance: Procurement, management, optimization, training and compliance.
b) Data Comprehension: Awareness, visibility and accessibility.
c) Real World Evidence (RWE) Generation: Analytics, biostatistics, deliverables, and communication (Figure 1).

Building the Internal Capability
Business units generating RWE within the life sciences have multiple objectives:
a) Hypothesis testing to answer key research questions.
b) Descriptive and exploratory studies for Hypothesis generation.
c) Rapid response analytics and competitive intelligence analytics.
d) Analytics to support clinical trial development and business development.
The impact of RWE is felt far and wide within the industry, aiding efforts in Market Access, Health Economics and Outcomes Research (HEOR), Medical Affairs, Marketing, Commercial, and Clinical Development. The Clinical Development frontier is of great interest to industry participants, as the FDA's published guidance on RWE for Regulatory decision making is due in the coming year and new analytical methods in predictive analytics and machine learning can improve and expedite the drug discovery process.
With these inherent opportunities, the logical goal for any knowledge-enabled organization is to develop and implement a long-term strategy for Data Governance, Comprehension and Analytics.
Data Governance
Data governance ensures the success of three critical components in data-driven organization support:
a) Development of data acquisition strategies to support strategic evidence needs,
b) Overseeing and ensuring data quality and fidelity, with quick resolution of possible quality conflicts.
c) Prompt reaction when new analytics requirements d) Possessing data but not understanding how to analyze emerge (Figure 2).

Data Governance offers a sound and resilient data foundation, minimizes errors, reduces processing time, and eliminates administrative overhead from all of the departments that use RWE.
Most importantly, central Data Governance permits the roll-out of Good Data Practice. Training for direct data users (data scientists & other business functions) and compliance with regulatory rules around patient anonymity result in more knowledgeable and productive users within the data hub. Acquiring, preparing, and deploying data is critical for organizations that are serious about RWE.
Data Comprehension
Data Comprehension leads to a better understanding of research initiatives, enables cooperation among data owners, promotes sharing of data resources, and minimizes the possibility of duplicating data acquisitions (Figure 3).
Implementation ofdata awareness, visibility, and accessibility will help solve perennial data problems experienced by different stakeholders within the organization. These problems include:
a) Possessing data and not knowing when to use it
b) Possessing data and not knowing what is available,
c) Possessing data and not knowing how it benefits the function, and
d) Possessing data but not understanding how to analyze

Addressing these through thorough Data Comprehension solves these problems, grows the knowledge pathway and provides the best return on your RWD investment.
RWE Generation
Establishing a working model for RWE is critical. A Data Hub Hybrid model includes both internal data scientists as well as external Analytic, Statistical, and Scientific support personnel. This Data Hub Hybrid offers numerous benefits:
a) Scalable analytics for strategic understanding of departmental needs
b) Comprehensive support, from research question development to programming and interpretation
c) Both the internal resources and external data science team work as one function with a service provider mentality.
d) Hybrid functional structure encourages cooperation on study development, delivery, and knowledge sharing, improving the collective performance of the group (Figure 4).
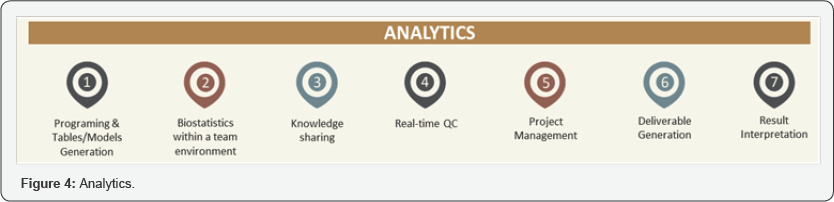
Measuring Success
Evidence Generation, Delivery and the degree of Confidence that other departments have in the deliverables of the Data and Analytics Hub is usually the Primary Measure of its success. However, there are empirical ways of measuring the success of the Data Hub and RWE working model as well, and these include:
a) Number of project collaborations between departments
b) Elimination of data source and study redundancies,
c) More available budget for functions to do non-RWE activities,
d) Increased number of publications.
With a rigorous methodology in place and the Data Hub operating on all cylinders, manufacturers can challenge the evidentiary requirements of today, while we build up to the exciting opportunities of tomorrow in artificial intelligence, machine learning, and predictive analytics.
For more articles in Open Access Journal of Case Studies please click on: https://juniperpublishers.com/jojcs/index.php
No comments:
Post a Comment